Upcoming Talks
Time:Wed., 15:30-16:30, Sept. 27, 2023
Venue:B627, Shuangqing Complex Building 双清B627
Speaker:Anqi Dong, University of California, Irvine
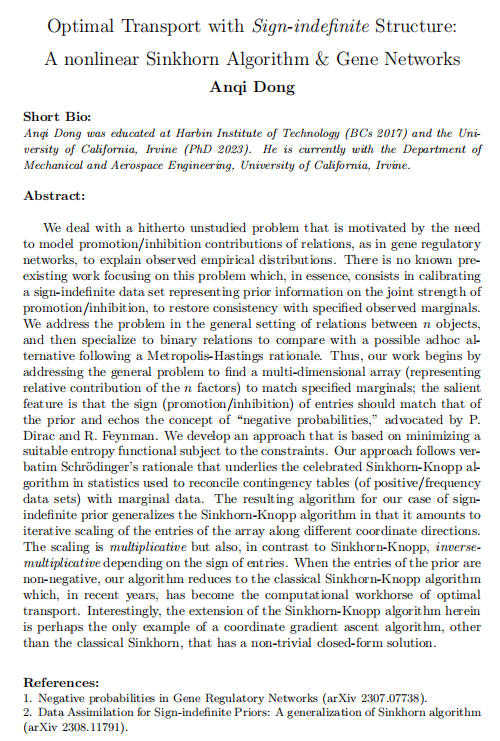
Past Talks
Title: Conservative, Positivity Preserving and Energy Dissipative Numerical Methods for the Poisson-Nernst-Planck Equations
Speaker: Zhongming Wang, Florida International University
Time: 10:00-11:00 on Thursday, March 23, 2023
Venue:Tencent Meeting: 394-2709-5975
Abstract:
We design and analyze some numerical methods for solving the Poisson-Nernst-Planck (PNP) equations. The numerical schemes, including finite difference method and discontinuous Glerkin method, respect three desired properties that are possessed by analytical solutions: I) conservation, II) positivity of solution, and III) free-energy dissipation. Advantages of different types of methods are discussed. Numerical experiments are performed to validate the numerical analysis. Modified PNP system that incorporating size and solvation effect is also studied to demonstrate the effectiveness of our schemes in solving realistic problems.
This is joint work with D. Jie, H. Liu, P. Yin, H. Yu and S. Zhou.
Bio:
Zhongming Wang, Ph.D., received his bachelor's degree in Computing Mathematics from City University of Hong Kong in 2003 and doctorate degree in Applied Mathematics from Iowa State University in 2008. He was a Postdoctoral Fellow at the University of California, San Diego from 2008 to 2011. He joined the Department of Mathematics and Statistics, Florida International University in 2011 and was promoted to associate professor in 2016.
Dr. Wang's research interests are in computational and applied mathematics. In particular, he has been working on
1. level set methods for high frequency wave propagations, two-phase flows and the Euler-Poisson equations;
2. direct Discontinuous Galerkin method for nonlinear Fokker-Planck equations
3. conservative, positivity preserving and energy dissipative numerical methods for the Poisson-Nernst-Planck equations.
Title: Certifiable Outlier-Robust Geometric Perception
Speaker:Heng Yang
Time:Fri., 10:00-11:00 am, Feb.24, 2023
Venue: Tencent Meeting ID: 740-508-035
Organizer: Chenglong Bao
Abstract:
I will present an overview of the theory of quantitative homogenization for elliptic and parabolic equations, with an emphasis on the "coarse-graining" point of view. I will begin by trying to motivate the results with some applications to models in mathematical physics. Time permitting, I will also discuss some open problems. Geometric perception is the task of estimating geometric models (e.g., object pose and 3D structure) from sensor measurements and priors (e.g., point clouds and neural network detections). The ubiquitous existence of outliers —measurements that tell no or little information about the models to be estimated— makes it theoretically intractable to perform estimation with guaranteed optimality. Despite this theoretical intractability, safety-critical robotics applications still demand trustworthiness and performance guarantees on perception algorithms.
In this talk, I present certifiable outlier-robust geometric perception, a new paradigm to design tractable geometric estimation algorithms that enjoy rigorous performance guarantees, i.e., they return an optimal estimate with a certificate of optimality for a majority of problem instances, but declare failure and provide a measure of suboptimality for worst-case instances. Particularly, I present two general-purpose algorithms in this paradigm: (i) an estimator that uses graph theory to prune gross outliers and leverages graduated non-convexity to compute the optimal model estimate with high probability of success, and (ii) a certifier that employs sparse semidefinite programming (SDP) relaxation and a novel SDP solver to endow the estimator with an optimality certificate or escape local minima otherwise. The estimator is fast and robust against up to 99% random outliers in practical perception applications, and the certifier can compute high-accuracy optimality certificates for large-scale problems beyond the reach of existing SDP solvers. I showcase certifiable outlier-robust perception on robotics applications such as scan matching, satellite pose estimation, and vehicle pose and shape estimation.
Speaker:
Heng Yang is an (incoming) Assistant Professor in the School of Engineering and Applied Sciences at Harvard University. He obtained his PhD from the Laboratory for Information and Decision Systems at MIT in 2022, M.S. at MIT in 2017, and B.Eng. at Tsinghua University in 2015. He is broadly interested in the algorithmic foundations of robot perception, decision-making, and learning, with particular focus on bringing large-scale convex optimization, semidefinite relaxation, statistics, and machine learning to safe and trustworthy autonomy. Heng Yang is a recipient of the Best Paper Award in Robot Vision at the 2020 IEEE International Conference on Robotics and Automation (ICRA), a Best Paper Award Honorable Mention from the 2020 IEEE Robotics and Automation Letters (RA-L), and a Best Paper Award Finalist at the 2021 Robotics: Science and Systems (RSS) conference.
Title: Solving high-speed flows using PINNs and DeepMMnets
Speaker: Zhiping Mao (Xiamen Univeristy)
Time: 2022/06/10, 10am-11am
Tencent Meeting ID:713-548-553
Join the meeting through the link: https://meeting.tencent.com/dm/1jTPXLWtFEK4
Abstract: Recently, neural network-based deep learning methods, which are different from the classical numerical methods, have attracted lots of attention not only in the traditional artificial intelligence community but also the scientific computing community. In this talk, I will introduce my work using physics-informed neural networks (PINNs) and deep multi-scale multi-physics nets (DeepMMnet) for high-speed flows. In particular, I shall solve the inverse problems of the shock wave problems in supersonic flow by using PINNs based on the information of density gradient and limited data of pressure and inflow conditions instead of using boundary conditions. Then I will introduce the inference of the flow past a normal shock in hypersonic flow by using the DeepMMnets with the help of DeepOnets.
Bio: Dr. Zhiping Mao is currently a Professor in School of Mathematical Science Xiamen University. He got his PhD degree in 2015 from Xiamen University. He then went to in Division of Applied Mathematics, Brown University to worked as Postdoc. He mainly interested in spectral methods and deep learning, and he published more then 20 SCI papers on top journal such as SIREV, SINUM, JCP, SISC, CMAME.
Title: Learning to Generate Data by Estimating Gradients of the Data Distribution
Speaker: Yang Song (Stanford University)
Time: 2022/06/10,11am-12pm
Tencent Meeting ID:713-548-553
Join the meeting through the link: https://meeting.tencent.com/dm/1jTPXLWtFEK4
Abstract: Generating data with complex patterns, such as images, audio, and molecular structures, requires fitting very flexible statistical models to the data distribution. Even in the age of deep neural networks, building such models is difficult because they typically require an intractable normalization procedure to represent a probability distribution. To address this challenge, I propose to model the vector field of gradients of the data distribution (known as the score function), which does not require normalization and therefore can take full advantage of the flexibility of deep neural networks. I will show how to (1) estimate the score function from data with flexible deep neural networks and efficient statistical methods, (2) generate new data using stochastic differential equations and Markov chain Monte Carlo, and even (3) evaluate probability values accurately as in a traditional statistical model. The resulting method, called score-based generative modeling, achieves record-breaking performance in applications including image synthesis, text-to-speech generation, time series prediction, and point cloud generation, challenging the long-time dominance of generative adversarial networks (GANs) on many of these tasks. Furthermore, unlike GANs, score-based generative models are suitable for Bayesian reasoning tasks such as solving ill-posed inverse problems, and I have demonstrated their superior performance on sparse-view computed tomography and accelerated magnetic resonance imaging.
Bio: Yang Song is a final year Ph.D. student at Stanford University. His research interest is in deep generative models and their applications to inverse problem solving and AI safety. His first-author papers have been recognized with an Outstanding Paper Award at ICLR-2021, and an oral presentation at NeurIPS-2019. He is a recipient of the Apple PhD Fellowship in AI/ML, and the J.P. Morgan PhD Fellowship.
Title:When does SGD favor generalizable solutions? A linear stability-based analysis
Speaker:Lei Wu,Peking University 吴磊(北京大学、数学科学学院)
Time:2022/06/03 15:00-16:00
Tencent Meeting ID:790-253-750
Join the meeting through the link:https://meeting.tencent.com/dm/QR7mPrp7kUBJ
Abstract:Deep learning models are often operated with far more unknown parameters than training examples. In such a case, there exist many global minima, but their test performances can be very different. Fortunately, stochastic gradient descent (SGD) can select the good ones without needing any explicit regularizations, suggesting certain "implicit regularization" at work. This talk will provide a quantitative explanation of this striking phenomenon from the perspective of linear stability. We prove that if a global minimum is linearly stable for SGD, then the flatness---as measured by the Hessian's Frobenius norm---must be bounded independently of the model size and sample size. Moreover, this flatness can bound the generalization gap of two-layer neural networks. Together, we show that SGD favors flat minima and flat minima provably generalize well. Note that these are made possible by exploiting the particular geometry-aware structure of SGD noise.
Bio:吴磊2021年入职北京大学数学学院,任助理教授;研究方向为深度学习的数学理论。他2012年获南开大学数学与应用数学专业学士学位,2018年毕业于北京大学计算数学专业,2018年11月至2021年10月先后在美国普林斯顿大学和宾夕法尼亚大学从事博士后研究。
Title: Cryo-Electron Microscopy Image Analysis: from 2D class averaging to 3D reconstruction
Speaker: Zhizhen Jane Zhao (UIUC)
Time:2022/05/27 10:00-11:00am Beijing Time
Tencent Meeting ID:536-366-394
Join the meeting through the link:https://meeting.tencent.com/dm/y7FgST4VVz7D
Abstract: Cryo-electron microscopy (EM) single particle reconstruction is an entirely general technique for 3D structure determination of macromolecular complexes. This talk focuses on the algorithms for 2D class averaging and 3D reconstruction for the single-particle images, assuming no conformation changes of the macromolecules. In the first part, I will introduce the multi-frequency vector diffusion maps to improve the efficiency and accuracy of cryo-EM 2D image classification and denoising. This framework incorporates different irreducible representations of the estimated alignment between similar images. In addition, we use a graph filtering scheme to denoise the images using the eigenvalues and eigenvectors of the MFVDM matrices. In the second part, I will present a 3D reconstruction approach, which follows a line of works starting from Kam (1977) that employs the autocorrelation analysis for the single particle reconstruction. At the end of the talk, I will briefly review the challenges and existing approaches for addressing the continuous heterogeneity in cryo-EM data.
Bio:Zhizhen Zhao is an Assistant Professor in the Department of Electrical and Computer Engineering at the University of Illinois at Urbana-Champaign. She joined University of Illinois in 2016. From 2014 to 2016, she was a Courant Instructor at the Courant Institute of Mathematical Sciences, New York University. She received the B.A. and M.Sc. degrees in physics from Trinity College, Cambridge University in 2008, and the Ph.D. degree in physics from Princeton University in 2013. She is a recipient of Alfred P. Sloan Research Fellowship (2020--2022). Her research interests include computational imaging, data science, and machine learning.
Title: Generalized Power Method for Generalized Orthogonal Procrustes Problem
Speaker: Shuyang Ling (New York University Shanghai)
Time:2022/05/20 10:00-11:00am Beijing Time
Tencent Meeting ID:817-714-783
Join the meeting through the link:https://meeting.tencent.com/dm/DzQ0iWQspYXN
Abstract: Given a set of multiple point clouds, how to find the rigid transformations (rotation, reflection, and shifting) such that these point clouds are well aligned?
This problem, known as the generalized orthogonal Procrustes problem (GOPP), plays a fundamental role in several scientific disciplines including statistics, imaging science and computer vision. Despite its tremendous practical importance, it is still a challenging computational problem due to the inherent nonconvexity. In this talk, we will discuss the semidefinite programming (SDP) relaxation of the generalized orthogonal Procrustes problems and prove that the tightness of the SDP relaxation holds, i.e., the SDP estimator exactly equals the least squares estimator, if the signal-to-noise ratio (SNR) is relatively large. We also prove that an efficient generalized power method with a proper initialization enjoys global linear convergence to the least squares estimator. In addition, we analyze the Burer-Monteiro factorization and show the corresponding optimization landscape is free of spurious local optima if the SNR is large. This explains why first-order Riemannian gradient methods with random initializations usually produce a satisfactory solution despite the nonconvexity. Our results resolve one open problem posed in [Bandeira, Khoo, Singer, 2015] on the tightness of the SDP relaxation in solving the generalized orthogonal Procrustes problem. Numerical simulations are provided to complement our theoretical analysis.
Bio: 凌舒扬现任职于上海纽约大学,是数据科学和数学方向的预聘制助理教授。在加入上海纽约大学之前,他于2017年至2019年在纽约大学柯朗数学研究所和数据科学研究所担任柯朗讲师。他在2017年6月从加州大学戴维斯分校应用数学专业获得博士学位,博士导师是Thomas Strohmer教授。他的研究兴趣主要在数据科学、信息科学、优化和信号处理等,主要成果发表在如Foundation of Computational Mathematics, Mathematical Programming, SIAM Journal on Optimization/Imaging Science, Applied and Computational Harmonic Analysis, IEEE Transactions on Information Theory, Journal of Machine Learning Research等杂志上。他的研究获得多项国家自然科学基金和科技部国家重点研发计划以及上海和国家人才计划的支持。
Title: Communication Compression in Distributed Learning
Speaker:Prof. Ming Yan (MSU) 严明(密歇根州立大学)
Time:2022/05/13 Fri. 10:00-11:00 am(Beijing Time)
Tencent Meeting ID:635-963-958
Join the meeting through the link:https://meeting.tencent.com/dm/8hAgdt0Ebh2C
Abstract:Large-scale machine learning models are trained by parallel (stochastic) gradient descent algorithms on distributed systems. The communications for gradient aggregation and model synchronization become the major obstacles for efficient learning as the number of computing nodes and the model's dimension scale up. In this talk, I will introduce several ways to compress the transferred data and reduce the overall communication such that the obstacles can be immensely mitigated. More specifically, I will introduce methods to reduce or eliminate the compression error without additional communication for both deterministic and stochastic algorithms.
Bio:Ming Yan is an associate professor in the Department of Computational Mathematics, Science and Engineering (CMSE) and the Department of Mathematics at Michigan State University. His research interests lie in computational optimization and its applications in image processing, machine learning, and other data-science problems. He received his B.S. and M.S in mathematics from University of Science and Technology of China in 2005 and 2008, respectively, and then Ph.D. in mathematics from University of California, Los Angeles in 2012. After completing his PhD, Ming Yan was a Postdoctoral Fellow in the Department of Computational and Applied Mathematics at Rice University from July 2012 to June 2013, and then moved to University of California, Los Angeles as a Postdoctoral Scholar and an Assistant Adjunct Professor from July 2013 to June 2015. He received a Facebook faculty Award in 2020.
Title: Normalizing field flow: solving forward and inverse stochastic differential equations using physics-informed flow model.
Speaker:Prof. Tao Zhou 周涛教授(中国科学院)
Time:2022/05/06 Fri. 16:00-17:00pm(Beijing Time)
Tencent Meeting ID:727-881-710
Join the meeting through the link:https://meeting.tencent.com/dm/uyr5e7URpGEz
Abstract:We introduce normalizing field flows (NFF) for learning random fields from scattered measurements. More precisely, we construct a bijective transformation between a reference random field (say, a Gaussian random field with the Karhunen-Lo\`eve (KL) expansion structure) and the target stochastic field, where the KL expansion coefficients and the invertible networks are trained by maximizing the sum of the log-likelihood on scattered measurements. The NFF model can be used to solve data-driven forward, inverse, and mixed forward/inverse stochastic partial differential equations in a unified framework. We demonstrate the capability of the proposed NFF model for learning non-Gaussian processes, mixed Gaussian processes, and forward \& inverse stochastic partial differential equations.
Bio:周涛,中国科学院数学与系统科学研究院研究员。曾于瑞士洛桑联邦理工大学从事博士后研究。主要研究方向为不确定性量化、随机最优控制以及时间并行算法等。在国际权威期刊如SIAM Review、SINUM、JCP等发表论文60余篇。2018年获自然科学基金委“优秀青年科学基金”资助。现担任SIAM J Sci Comput、Commun. Comput. Phys、J Sci Comput等国际期刊编委,国际不确定性量化期刊(International Journal for UQ)副主编。
Title: Blind Image Deblurring: Past, Current and Future
Speaker:曾铁勇教授(香港中文大学)
Time:2022/04/29 Fri. 10:00-11:30am(Beijing Time)
Tencent Meeting ID:714-321-553
Join the meeting through the link:https://meeting.tencent.com/dm/pqQawNfO7sIl
Abstract:Blind image deblurring is a challenging task in imaging science where we need to estimate the latent image and blur kernel simultaneously. To get a stable and reasonable deblurred image, proper prior knowledge of the latent image and the blur kernel is urgently required. In this talk, we address several of our recent attempts related to image deblurring. Indeed, different from the recent works on the statistical observations of the difference between the blurred image and the clean one, we first report the surface-aware strategy arising from the intrinsic geometrical consideration. This approach facilitates the blur kernel estimation due to the preserved sharp edges in the intermediate latent image. Extensive experiments demonstrate that our method outperforms the state-of-the-art methods on deblurring the text and natural images. Moreover, we discuss the Quaternion-based method for color image restoration. After that, we extend the quaternion approach for blind image deblurring, and discuss the pixel correction method.
Bio: Dr. Tieyong Zeng is a Professor at the Department of Mathematics, The Chinese University of Hong Kong (CUHK). Together with colleagues, he has founded the Center for Mathematical Artificial Intelligence (CMAI) since 2020 and served as the director of CMAI. He received the B.S. degree from Peking University, Beijing, China, the M.S. degree from Ecole Polytechnique, Palaiseau, France, and the Ph.D. degree from the University of Paris XIII, Paris, France, in 2000, 2004, and 2007, respectively. His research interests include image processing, optimization, artificial intelligence, scientific computing, computer vision, machine learning, and inverse problems. He has published around 100 papers in the prestigious journals such as SIAM Journal on Imaging Sciences, SIAM Journal on Scientific Computing, Journal of Scientific Computing, IEEE Transactions on Pattern Analysis and Machine Intelligence (TPAMI), International Journal of Computer Vision (IJCV), IEEE Transactions on Neural Networks and Learning Systems (TNNLS), IEEE Transactions on Image Processing (TIP), IEEE Medical Imaging (TMI), and Pattern Recognition.
Title: An Algebraically Converging Stochastic Gradient Descent Algorithm for Global Optimization
Speaker:杨雨楠(ETH Zurich)
Time:2022/04/15 Fri. 16:30-17:30pm(Beijing Time)
Tencent Meeting ID:513-143-699
Join the meeting through the link:https://meeting.tencent.com/dm/UVd6JH1EObcy
Abstract:We propose a new stochastic gradient descent algorithm for finding the global optimizer of nonconvex optimization problems, referred to here as ``AdaVar''. A key component in the algorithm is the adaptive tuning of the randomness based on the value of the objective function. In the language of simulated annealing, the temperature is state-dependent. With this, we can prove global convergence with an algebraic rate both in probability and in the parameter space. This is a major improvement over the classical rate from using a simpler control of the noise term. The convergence proof is based on the actual discrete setup of the algorithm. We also present several numerical examples demonstrating the efficiency and robustness of the algorithm for global convergence.
Bio:Yunan Yang is an applied mathematician working in inverse problems and optimal transport. Currently, Yunan is an advanced Fellow at the Institute for Theoretical Studies at ETH Zurich. She will be a Tenure-Track Assistant Professor in the Department of Mathematics at Cornell University starting in July 2023. Yunan Yang earned a Ph.D. degree in mathematics from the University of Texas at Austin in 2018, supervised by Prof. Bjorn Engquist. From September 2018 to August 2021, Yunan was a Courant Instructor at the Courant Institute of Mathematical Sciences, New York University.
Title: Algorithms for Joint Community Detection and Synchronization
Speaker:Zhizhen Jane Zhao(UIUC)
Time:2022/04/08 Fri. 10:00-11:30am(Beijing Time)
Tencent Meeting ID:941-679-826
Join the meeting through the link:https://meeting.tencent.com/dm/QNdkFcTHaIHC
Abstract:In the presence of heterogeneous data, where randomly rotated objects fall into multiple underlying categories, it is challenging to simultaneously classify them into clusters and synchronize them based on pairwise relations. This gives rise to the joint problem of community detection and synchronization. We introduce a probabilistic model that extends the celebrated stochastic block model to this new setting where both orthogonal transformation and cluster identities are to be determined. To solve the corresponding non-convex problem, we introduce two types of relaxations. The first approach is based on semidefinite relaxation, and we introduce new matrix concentration inequalities to analyze the conditions for exact recovery. The second approach is based on the spectral relaxation. The algorithm consists of three simple steps: a spectral decomposition followed by a blockwise column pivoted QR factorization, and a step for cluster assignment and group element recovery. We leverage the `leave-one-out' technique to establish a near-optimal guarantee for exact recovery of the cluster memberships and stable recovery of the orthogonal transforms. We will show numerical results to demonstrate the efficacy of our proposed algorithms.
Bio: Zhizhen Zhao is an Assistant Professor in the Department of Electrical and Computer Engineering at the University of Illinois at Urbana-Champaign. She joined University of Illinois in 2016. From 2014 to 2016, she was a Courant Instructor at the Courant Institute of Mathematical Sciences, New York University. She received the B.A. and M.Sc. degrees in physics from Trinity College, Cambridge University in 2008, and the Ph.D. degree in physics from Princeton University in 2013. She is a recipient of Alfred P. Sloan Research Fellowship (2020--2022). Her research interests include computational imaging, data science, and machine learning.
Title: Numerical methods for nonlocal models: asymptotically compatible schemes and multiscale modeling
Speaker:田小川(UCSD)
Time:2022/04/01 Fri. 10:00-11:30am(Beijing Time)
Tencent Meeting ID:731-149-400
Join the meeting through the link:https://meeting.tencent.com/dm/WJ78UcwJd7ib
Abstract:Nonlocal continuum models are in general integro-differential equations in place of the conventional partial differential equations. While nonlocal models show their effectiveness in modeling a number of anomalous and singular processes in physics and material sciences, for example, the peridynamics model of fracture mechanics, they also come with increased difficulty in computation with nonlocality involved. In this talk, we will give a review of the asymptotically compatible schemes for nonlocal models with a parameter dependence. Such numerical schemes are robust under the change of the nonlocal length parameter and are suitable for multiscale simulations where nonlocal and local models are coupled. We will discuss finite difference, finite element and collocation methods for nonlocal models as well as the related open questions for each type of the numerical methods.
Title: Fast solvers based on integral equation methods for wave scattering and inverse scattering
Speaker: 赖俊 (浙江大学)
会议时间:2022/03/18 10:00 中国标准时间 - 北京
#腾讯会议:584-263-066
Abstract: Wave scattering and inverse scattering have been appeared in a lot of important applications, including non-destructive testing, seismic inversion, radar technology and medical imaging, etc. Integral equation method provides an effective tool for solving wave scattering and inverse scattering problems. In this talk, fast and high order numerical methods based on integral equations will be presented for elastic wave equations. In particular, I will talk about the numerical algorithms using high order discretization of singular integrals and the fast multipole method for evaluating the elastic wave scattering in complex media, as well as their applications in inverse elastic wave scattering problems with phaseless scattered data and the imaging of multiple elastic particles.
赖俊,南京大学数学系本科,美国密西根州立大学应用数学博士毕业,曾任纽约大学柯朗数学研究所博士后及讲师(Courant Instructor),目前任浙江大学数学科学学院“百人计划”研究员,主要研究声波,电磁波及弹性波方程的散射与反散射问题,在数学知名杂志ACHA,SISC,Math Comp,Inverse Problems等发表文章多篇,主持国家面上项目,并参与基金委重大研究计划,基金委创新群体等研究,曾入选中组部高层次青年人才计划,获十一届全国反问题年会“优秀青年学术奖”。
Title: Feature Space Fusion for Heterogeneous Scattered Data
Time:2022/03/11 Fri. 16:00-17:00
Venue:理科楼数学系A404会议室
Abstract: Scattered data are data collected and stored individually at local data centers. Unlike distributed data, a popular notion in distributed learning, scattered data can be highly heterogeneous. Specifically, scattered data usually violate two common assumptions for distributed data, i.e., across all data centers, (1) the predictors follow the same distribution, and (2) the response depends on the predictors through the same link function. Classical feature fusion approaches that are widely used for distributed data analysis may have deteriorating performance when either or both assumptions are not met. We develop a feature space fusion framework for scattered data without requiring these two assumptions. The proposed framework is built upon the multi-index model. We assume the data w.r.t. different data centers share a universal informative feature subspace, while the response in each center depends on the subspace through a center-specific unknown link function. We propose an algorithm called ``SUFFicient Universal Subspace Extraction" (SUFFUSE) to estimate such a subspace. Theoretically, we show the estimated subspace is consistent under some mild regularity conditions. The asymptotic normality of the estimator is also established. Numerical studies on various synthetic and real-world datasets demonstrate the superior performance of the proposed method in comparison with mainstream competitors.
Speaker: 张静怡
Profile: 2011年毕业于武汉大学;2013年于武汉大学获得统计学硕士学位;2020年于美国佐治亚大学获得统计学博士学位,师从钟文瑄教授与马平教授。2020年开始在清华大学统计学研究中心担任助理教授。主要研究方向为数据融合,去中心化计算,差分隐私和最优传输理论。
Title: A class of second-order geometric quasilinear hyperbolic PDEs and their applications
Time:2022/03/04 10:00-11:30am (Beijing Time)
Tencent Meeting ID:659-408-687
Speaker: Guozhi Dong
Abstract: Motivated by applications in mathematical imaging and recent advances of second-order dynamics in optimization, we consider a class of second-order quasilinear hyperbolic PDEs. We focus on in particular the second-order counterparts of the total variation flow and the mean curvature flow for level sets of scalar functions in this talk. Analytical results as well as some numerical behavior of solutions will be presented. Some open issues and potential extensions will be discussed in the end.
Profile: Dr. Guozhi Dong is a research scientist at the Institute for Mathematics, Humboldt University of Berlin. He is also affiliated to Weierstrass Institute for Applied Analysis and Stochastics (WIAS). He obtained his Bachelor degree at Hunan Normal University in 2007, and then worked there as a secretary of research affairs for 5 years. From 2012 to 2017, he worked as a research assistant at the University of Vienna, and obtained his PhD degree. His research interests include inverse and imaging problems, optimization with PDE constraints, and numerical methods for PDEs, in particular for PDEs defined on manifolds.
Join the meeting through the link:https://meeting.tencent.com/dm/hBS4GXd04yri
题目: 随机矩阵与矩阵集中不等式
报告人: 黄德 (北京大学)
时间: 2022-02-25 星期五 10:00-11:00am
地点: 宁斋W11
摘要: 随机矩阵理论,对于现代诸多随机算法的理论推导有着极其深刻的意义。而矩阵集中不等式,则从概率的角度刻画了随机矩阵偏离其期望的程度,是随机矩阵理论在实际应用中的主要表现形式,为随机算法的收敛性提供了有效的理论保障。作为经典标量集中不等式的推广,矩阵集中不等式是测度集中性在高维复杂代数结构中的反映。 一些关于基本随机矩阵模型的集中不等式,在计算数学和大数据科学中已经有着广泛应用。然而,许多经典的关于更为复杂概率模型的标量集中不等式仍未被合理地推广到矩阵范畴;其主要困难在于矩阵本身作为线性算子的不可交换性。本次报告主要介绍随机矩阵领域,特别是矩阵集中不等式领域的基本情况和当前研究热门。报告将从测度的集中性出发,从标量集中不等式引入矩阵集中不等式,并通过一些典型结果的简要证明,展现不可交换性在集中不等式推导过程带来的主要困难和挑战,以及针对这些困难所建立的有效理论方法体系。 报告也将介绍近年来随机矩阵领域的一些典型的理论发展和实际应用,如通过 Markov 半群理论建立非线性矩阵集中不等式等;同时罗列一些尚未被解决的重要问题,如非交换 Rosenthal 不等式猜想等。
报告人简介:
个人经历
2022-至今 北京大学数学科学学院 助理教授
2021-2022 美国加州理工学院 博士后
2021 美国加州理工学院 应用数学博士
2015 北京大学 物理双学位
2015 北京大学数学科学学院 学士
研究兴趣:
随机矩阵和矩阵集中不等式;偏微分方程数值解;流体力学方程奇异解
Title: Error Bounds for Deep Estimation Allowing Overparametrization
Time: 2022/02/18 10:00-12:00 am.
Tencent Meeting ID:286-772-015
Speaker: 焦雨领 (数学与统计学院,武汉大学)
Join the meeting through the link:https://meeting.tencent.com/dm/ZM7iglJy94GD
Abstract: The three errors in deep learning(approximation error, statistical (generalization) error, optimization error) are not compatible due to overparametrization. In this talk, I will present a new analysis for deep estimation which may break this dilemma.The key point is approximation with norm control. We derive error bounds for deep regression and GANs allowing overparametrization. As an application, we provide theoretical analysis for generative learning with Schr {\" o} dinger Bridge.
Title: From ODE Solvers to Accelerated Optimization Methods
Time: 2022/01/21 08:30-10:00 am.
Tencent Meeting ID:904-824-431 Passcode:220121
Speaker: Prof. Chen Long (Department of Mathematics, University of California, Irvine)
Join the meeting through the link:https://meeting.tencent.com/dm/ANYcbo1Rzl2d
Abstract: Convergence analysis of accelerated first-order methods for convex optimization problems are presented from the point of view of ordinary differential equation (ODE) solvers. We first take another look at the acceleration phenomenon via A-stability theory for ODE solvers and present an explanation by transformation of spectrum to the complex plane. After that, we present the Lyapunov framework for dynamical system and introduce the strong Lyapunov condition. Many existing continuous convex optimization models, such as gradient flow, heavy ball system, Nesterov accelerated gradient flow, and dynamical inertial Newton system etc, are addressed and analyzed in this framework.
This is a joint work with Dr. Hao Luo at Peking University.
Title: An efficient unconditionally stable method for Dirichlet partitions in arbitrary domains
Time:2022/01/14 10:00-11:30 am.
Speaker:王东老师
Tencent meeting ID:819-151-291 Passcode: 220114
You can also join in the meeting through this link: https://meeting.tencent.com/dm/JdcIcbpSXKF3
Abstract:
A Dirichlet k-partition of a domain is a collection of k pairwise disjoint open subsets such that the sum of their first Laplace--Dirichlet eigenvalues is minimal. In this talk, we propose a new relaxation of the problem by introducing auxiliary indicator functions of domains and develop a simple and efficient diffusion generated method to compute Dirichlet k-partitions for arbitrary domains. The method only alternates three steps: 1. convolution, 2. thresholding, and 3. projection. The method is simple, easy to implement, insensitive to initial guesses and can be effectively applied to arbitrary domains without any special discretization. At each iteration, the computational complexity is linear in the discretization of the computational domain. Moreover, we theoretically prove the energy decaying property of the method. Experiments are performed to show the accuracy of approximation, efficiency, and unconditional stability of the algorithm. We apply the proposed algorithms on both 2- and 3-dimensional flat tori, triangle, square, pentagon, hexagon, disk, three-fold star, five-fold star, cube, ball, and tetrahedron domains to compute Dirichlet k-partitions for different k to show the effectiveness of the proposed method. Compared to previous work with reported computational time, the proposed method achieves hundreds of times acceleration.
Profile:
王东博士现在是香港中文大学(深圳)的助理教授。他的主要研究兴趣包括计算流体力学、计算材料科学、图像处理、及机器学习。王东博士于2013年在四川大学取得数学的学士学位,于2017年在香港科技大学取得应用数学博士学位。在2020年8月加入香港中文大学(深圳)之前,他曾在美国犹他大学数学系任助理教授讲师。
Title:Massive Random Access for 5G and Beyond: An Optimization Perspective
Time:2021/12/10 4pm-5pm
腾讯会议号:414-425-827
地点:近春园西楼3层报告厅
Abstract:Massive access, also known as massive connectivity or massive machine-type communication (mMTC), is one of the three main use cases of the fifth-generation (5G) and beyond 5G (B5G) wireless networks defined by the International Telecommunication Union. Different from conventional human-type communication, massive access aims at realizing efficient and reliable communications for a massive number of Internet of Things (IoT) devices. The main challenge of mMTC is that the BS can efficiently and reliably detect the active devices based on the superposition of their unique signatures from a large pool of uplink devices, among which only a small fraction is active. In this talk, we shall present some recent results of massive access from an optimization perspective. In particular, we shall present optimization formulations and algorithms as well as some phase transition analysis results.
个人简介:刘亚锋,2007年毕业于西安电子科技大学理学院数学系,2012年在中国科学院数学与系统科学研究院获得博士学位(导师:戴彧虹研究员);博士期间,受中国科学院数学与系统科学研究院资助访问明尼苏达大学一年(合作导师:罗智泉教授)。博士毕业后,他一直在中国科学院数学与系统科学研究院计算数学所工作,2018年晋升为数学与系统科学研究院副研究员。他的主要研究兴趣是最优化理论与算法及其在信号处理和无线通信等领域中的应用。曾获2011年国际通信大会“最佳论文奖”,2018年数学与系统科学研究院“陈景润未来之星”,2018年中国运筹学会“青年科技奖”,2020年IEEE通信学会亚太地区“杰出青年学者奖”等。他目前担任《IEEE Transactions on Wireless Communications》、《IEEE Signal Processing Letters》和《Journal of Global Optimization》期刊的编委。他是IEEE信号处理学会SPCOM(Signal Processing for Communications and Networking)的技术委员会成员。他的工作获得国家自然科学基金委青年基金、面上项目和优秀青年基金的资助。
[Title]A2DR: Open-Source Python Solver for Prox-Affine Distributed Convex Optimization
Date: 2021.08.19 (10am-11am in Beijing Time Zone)
Tencent meeting: 839333395
Speaker: Junzi Zhang (Applied Scientist, Amazon)
Short Bio:Junzi Zhang is currently working at Amazon Advertising as an Applied Scientist. He got his Ph.D. degree in Computational Mathematics at Stanford University, advised by Prof. Stephen P. Boyd from Stanford Department of Electrical Engineering. He has also been working closely with Prof. Xin Guo and Prof. Mykel J. Kochenderfer. Before coming to Stanford, he obtained a B.S. degree in applied mathematics from School of Mathematical Sciences, Peking University, where he conducted his undergraduate research under the supervision of Prof. Zaiwen Wen and Prof. Pingwen Zhang. His research has been focused on the design and analysis of optimization algorithms and software, and extends broadly into the fields of machine learning, causal inference and decision-making systems (especially reinforcement learning). He is also recently extending his research to federated optimization, predictive modeling and digital advertising. His research had been partly supported by Stanford Graduate Fellowship. More information can be found on his personal website at https://web.stanford.edu/~junziz/index.html.
Abstract:We consider the problem of finite-sum non-smooth convex optimization with general linear constraints, where the objective function summands are only accessible through their proximal operators. To solve it, we propose an Anderson accelerated Douglas-Rachford splitting (A2DR) algorithm, which combines the scalability of Douglas-Rachford splitting and the fast convergence of Anderson acceleration. We show that A2DR either globally converges or provides a certificate of infeasibility/unboundedness under very mild conditions. We describe an open-source implementation (https://github.com/cvxgrp/a2dr) and demonstrate its outstanding performance on a wide range of examples. The talk is mainly based on the joint work [SIAM Journal on Scientific Computing, 42.6 (2020): A3560–A3583] with Anqi Fu and Stephen Boyd.
题目:Landscape analysis of non-convex optimizations in phase retrieval
报告人: 蔡剑锋,香港科技大学
时间: 2020-07-17, 10:00-11:00 AM
方式: ZOOM
会议ID: 13320196942
摘要:Non-convex optimization is a ubiquitous tool in scientific and engineering research. For many important problems, simple non-convex optimization algorithms often provide good solutions efficiently and effectively, despite possible local minima. One way to explain the success of these algorithms is through the global landscape analysis. In this talk, we present some results along with this direction for phase retrieval. The main results are, for several of non-convex optimizations in phase retrieval, a local minimum is also global and all other critical points have a negative directional curvature. The results not only will explain why simple non-convex algorithms usually find a global minimizer for phase retrieval, but also will be useful for developing new efficient algorithms with a theoretical guarantee by applying algorithms that are guaranteed to find a local minimum.
题目: 针对训练测试数据偏差的鲁棒深度学习方法初探
报告人: 孟德宇教授,西安交通大学
时间: 2020-7-10, 9:00-10:00 AM
方式: 腾讯会议
会议 ID: 304 179 559
摘要: 在现实复杂环境下,用以训练的数据标记通常包含大量噪声(错误标记)。采用数据加权的方式是对该噪声标记问题一种通用的方法,例如侧重于易分类样本的自步学习方法与侧重于难分类样本的boosting算法等。然后,目前对数据加权仍然缺乏统一的学习模式,且一般总要涉及超参数选择的问题。本报告讲汇报一种新的元学习方法,通过在无偏差元数据的引导下,能够对存在偏差的噪声标记数据的训练模式进行有效的调节与控制,从而在很大程度上避免了超参数调节的问题,并通过数据驱动的方式实现了自适应选择权重赋予的方式。通过在各种包含异常标注数据集上的测试,初步验证了该方法的有效性与稳定性。
报告人简介: 孟德宇,西安交通大学教授,博导,任西安交大大数据算法与分析技术国家工程实验室机器学习教研室负责人。主要研究兴趣为机器学习、计算机视觉与人工智能的基础研究问题。共发表论文100余篇,其中IEEE Trans.长文36篇, CCF A类会议论文37篇。
题目: The power of depth in deep Q-Learning
报告人: 林绍波教授,西安交通大学
时间: 2020-7-10, 10:00-11:00 AM
方式:腾讯会议
会议 ID: 304 179 559
Abstract: With the help of massive data and rich computational resource, deep Q-learning has been widely used in operations research and management science and receives great success in numerous applications including, recommender system, games and robotic manipulation. Compared with avid research activities in practice, there lack solid theoretical verifications and interpretability for the success of deep Q-learning, making it be a little bit mystery. The aim of this talk is to discuss the power of depth in deep Q-learning. In the framework of statistical learning theory, we rigorously prove that deep Q-learning outperforms the traditional one by showing its good generalization error bound. Our results shows that the main reason of the success of deep Q-learning is due to the excellent performance of deep neural networks (deep nets) in capturing special properties of rewards such as the spatially sparse and piecewise constant rather than due to their large capacities. In particular, we provide answers to questions why and when deep Q-learning performs better than the traditional one and how about the generalization capability of deep Q-learning.
报告人简介: 林绍波:西安交通大学教授、博导。研究方向为分布式学习理论、深度学习理论及强化学习理论。主持或以核心成员参与国家自然科学基金9项.在JMLR, ACHA, IEEE-TSP, SIAM-JNA 等著名期刊发表论文60余篇。