Learning outcomes:
A student who has taken this course will:
show an in-depth knowledge of the potential outcomes framework for causal inference,
show an in-depth understanding of assumptions underlying causal analyses with experimental and observational data,
master theory presented for estimation of causal parameters in randomized experiments and observational studies,
master the application of parametric and non/semiparametric estimators of causal effects presented in the course,
be able to perform sensitivity analyses on estimates of a causal effect.
Content:
Potential outcomes, theory and assumptions
Fisher's exact test, Neymans approach to completely randomized experiments and model based (Bayesian) inference
Causal effect estimators, propensity score - model building, stratification, matching and weighing
Variance estimation
Instrumental variables
Instruction:
Instruction is given in the form of in-class lectures. Computer exercises/assignments will be distributed. Solutions are to be presented by students and discussed in the class (Discuss).
Book:
Imbens Guido W. and Donald B. Rubin (IR), Causal inference in statistics, social, and biomedical sciences, Cambridge University Press.
Preliminary schedule:
Monday 24/6 10-12 Introduction 13-15 Chapter 3 IR
Tuesday 25/6 10-12 Chapter 4 IR 13-15 Chapter 5 IR
Wednesday 26/6 10-12 Chapter 6 IR 13-15 Chapter 7 IR
Thursday 27/6 10-12 Chapter 8 IR 13-15 Chapter 9 IR
Friday 28/6 10-12 Discuss
Monday 1/7 10-12 Chapter 11 IR 13-15 Chapter 12 IR
Tuesday 2/7 10-12 Chapter 13 IR 13-15 Chapter 14 IR
Wednesday 3/7 10-12 Chapter 15 IR 13-15 Chapter 16 IR
Thursday 4/7 10-12 Chapter 17 IR 13-15 Chapter 18 IR
Friday 5/7 10-12 Discuss
Monday 8/7 10-12 Chapter 19 IR 13-15 Chapter 20 IR
Tuesday 9/7 10-12 Chapter 21 IR 13-15 Chapter 22 IR
Wednesday 10/7 10-12 Discuss 13-15 Chapter 23 IR
Thursday 11/7 10-12 Chapter 24 IR 13-15 Chapter 25 IR
Friday 12/7 10-12 Discuss
清华大学双清综合楼A座地址:北京市海淀区逸清南路西延6号院1号
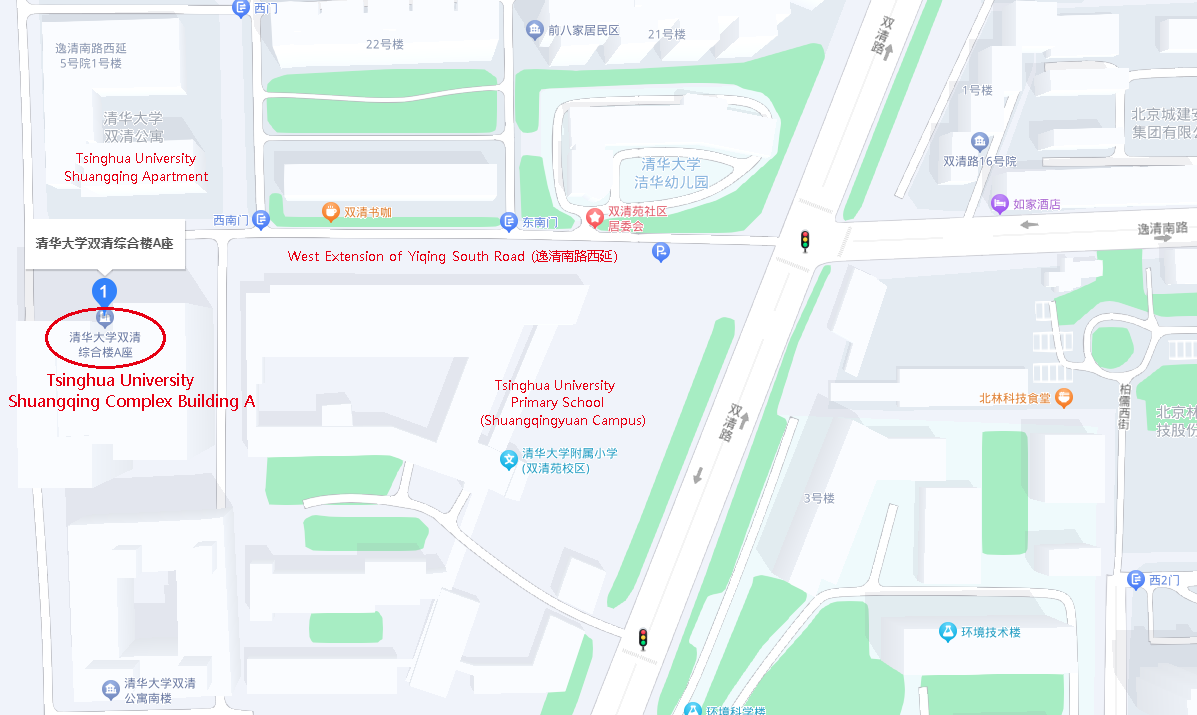